What if Michael Faraday Used Machine Learning?
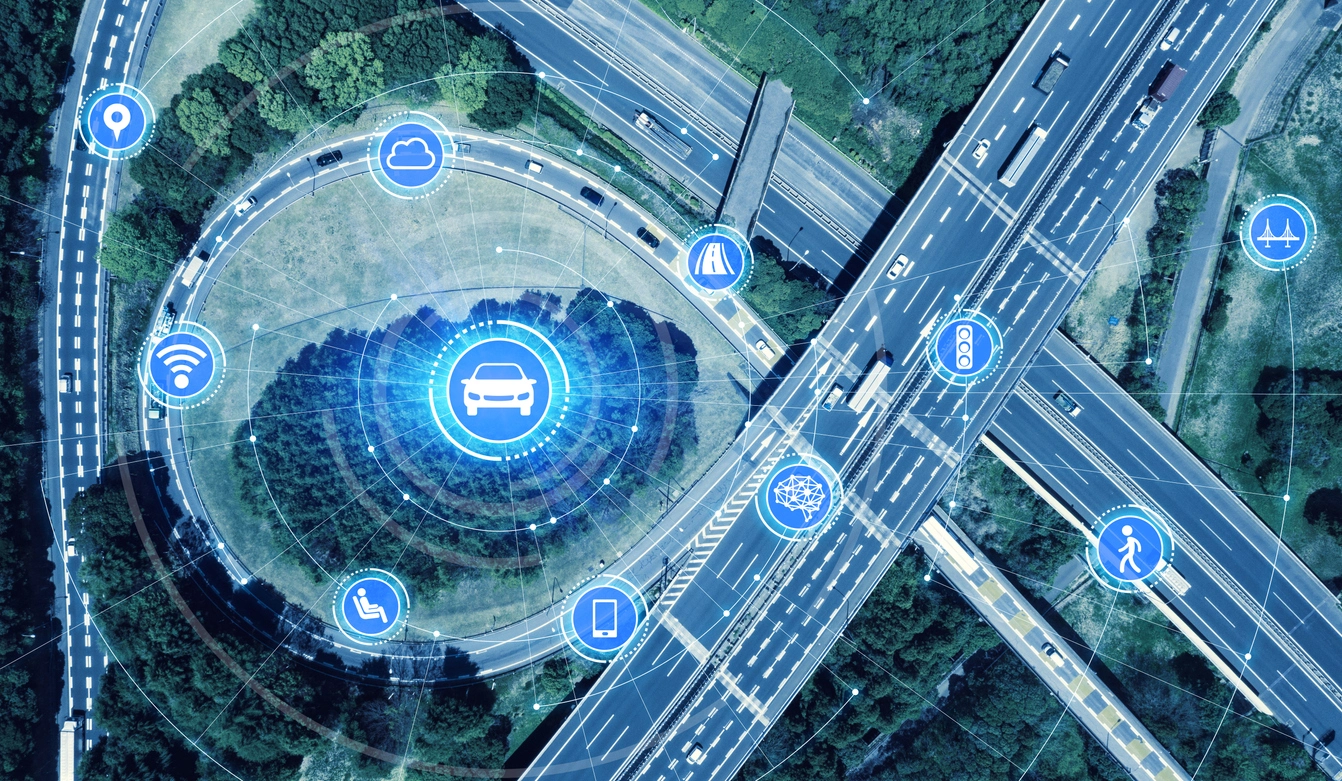
One of the most transformative scientific discoveries of the 19th century is the development of “lines of forces” in electromagnetism. It was widely believed that electricity and magnetism, originally modeled after gravity, acted instantaneously across a distance through a “fluid.” Electrical forces required an electrical fluid; magnetic forces required a magnetic fluid!
The experimental data was very compelling and fit well into equations developed by the leading scientists of the era including Charles-Augustinde Coulomb, André Marie Ampère, and Hans Christian Oersted, whose names are affixed to fundamental units of electromagnetic properties. It seemed that theory, models and data were axiomatic. But were they?
To Michael Faraday who was not skilled in mathematics and was an outsider to the Newtonian school, the explanations seemed intuitively wrong. Faraday, a prolific experimentalist, proceeded over the course of over thirty years to experiment with both electricity and magnetism. He demonstrated magnetic lines of forces by sprinkling iron filings on paper over a magnet. His intuition and insights led to the discovery of several principles of electrical engineering and electrochemistry, including electric induction, electrolysis and many others. It was James Clerk Maxwell, decades later as the consummate mathematician, who studied Faraday’s meticulously written papers to formulate his four eponymous equations, and in the process rewrote the classical theory of electromagnetism.
Machine Learning (ML), broadly speaking, has unleashed a gigantic technological capability to parse through large data sets and develop in machines human-like attributes. As I was recently reading the biographies of Faraday and Maxwell, it left me wondering a simple yet vexing question: “What if ML were available to scientists of the 19thcentury?”
One could argue that experiments conducted by scientists preceding Faraday and their corresponding data sets would constitute fertile training ground for modern ML engines. Much as the scientists were trained in the Newtonian school, their data were, too, biased by Newtonian philosophies. Would it be fair to expect that ML engines reach similar conclusions of the era, and thus miss Faraday’s intuition?
Electromagnetism and other branches of science are governed by fundamental physical laws that are verified with experimental observations. The application of these laws is a fundamental pillar of engineering. Batteries are subject to scientific laws and can be represented with physical models. Replacing physics with ML would not be the wisest use of this new technological tool. After all, collecting large data sets from electrical motors will not change Faraday’s law of induction. By the same token, collecting large datasets on batteries will not change the physical principles and equations that govern the battery.
If you are an ardent ML advocate and I am causing you fury or angst, please be patient with me for one more moment. Batteries are engineering wonders. They do follow physical laws but they are also manufactured in complex factories and used by humans. It is the manufacturing variability and field usage patterns where ML contributes to our knowledge. This observation allows us to divide battery know-how, including diagnostics, efficiency and optimization, into two distinct categories:
a) A first category that allows us to measure, characterize and optimize physical attributes of the battery using accurate physical models. For instance, measuring and determining the factors that cause loss of battery capacity, or identifying the early onset of metallic lithium dendrites are best left to the realm of able physical models. Here we can optimize the battery’s ability to accept fast charging by placing intelligent models at the edge, meaning in the immediate vicinity of the battery, and avoiding latency problems and costs of transmitting large data sets;
b) A second category that leverages ML capabilities to analyze manufacturing variances and widely differing usage patterns. Here, for example, we can optimize for charging and driving behaviors for vehicles and fleets, determine when to safely bring a vehicle in for battery maintenance, or establish a residual value of a battery.
If in part (a) we can enable an electric vehicle to charge safely and reliably using a 350-kW charging station, it is in part (b) where we can optimize the charging patterns for a fleet of taxis. If in part (a) we can estimate with high confidence a vehicle’s power and energy capabilities in the present and the future, it is in part (b) where we can identify trucks in a fleet that are most suited to complete a particular delivery route.
The genius of ML is to recognize where best to deploy this tool. The combination of human ingenuity aided by ML capabilities is the catalyst for accelerating innovation. It is then that we can rest comfortably in the notion that no ML could ever have replaced Michael Faraday, but also realize that the question was ill-posed from the very beginning...