THE ROLE OF BATTERY ANALYTICS – PART II
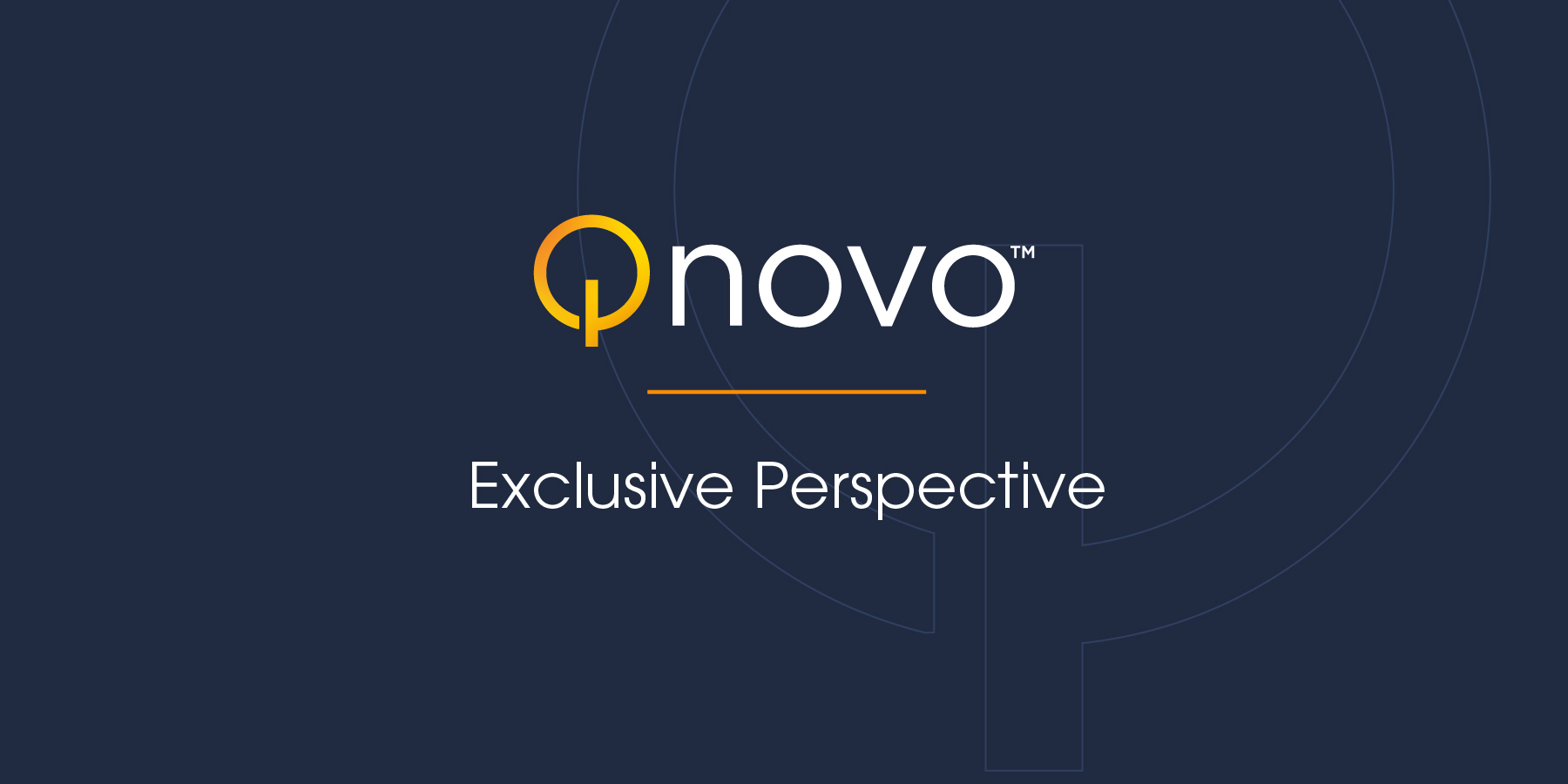
The lithium-ion battery should be a simple device. After all, it has only two terminals: one positive and another negative. Yet, this statement can’t be farther from the truth!
The operation of a lithium-ion battery is governed by fundamental scientific principles – many of them covering physics and electrochemistry (that’s the science tying electricity and chemistry). For instance, the basic energy storage mechanism discussed in Part I relies on the diffusion of lithium ions into the anode – sometimes known as Fick’s law in physics. The dependence of many of the battery’s properties on temperature follows specific scientific principles such as the Arrhenius equation. In other words, the battery’s functionality and aging are subject to deterministic equations – not a set of random processes.
Naturally, differences in manufacturing and in usage patterns reflect as statistical deviations in functionality and aging. For instance, process variations in the manufacturing of the anode will result in variability in the temperature coefficients of the battery’s structural materials. Hence, an effective approach to understanding the battery’s behavior combines both fundamental scientific principles and targeted statistical data analysis.
Fundamental scientific principles lend themselves to models. Models are helpful in science - they help us understand what goes under the hood to optimize the battery’s functionality, as well as make predictions, or estimates, of future functionality. Historically, engineers modeled batteries as equivalent electrical circuits – a combination of resistors, capacitors and even some coil inductors. Such models can be effective, but they neglect to consider non-linear physical and electrochemical processes within the battery. For instance, the formation of lithium metal dendrites (see Part I) is a highly non-linear electrochemical process that is poorly modeled using circuit elements. Consequently, understanding aging and failure requires a separate set of electrochemical models, i.e., models that capture the physics and electrochemistry within a lithium-ion battery, often in three-dimensions (3D), as batteries are not two-dimensional devices.
Electrochemical models alone are also insufficient – statistical data science is an integral complement to evaluate the effect of manufacturing and usage variabilities on the battery’s behavior. Outputs of models are only as good as inputs to these models. These inputs are derived from external measurements that reflect certain properties of the battery including electrical measurements (e.g., voltage, current, temperature, frequency…etc.) or other types of measurements.
An easy analogy is weather forecasting. Weather is governed by physics – more specifically thermodynamics. Sophisticated supercomputer models generate representative weather maps on a global scale. But these models depend on inputs derived from an extensive network of global sensors (e.g., temperature, pressure, solar flux…etc.). Weather forecasting fails in the absence of these sensors.
One established measurement method is called Electrochemical Impedance Spectroscopy (EIS). It has been a workhorse in electrochemical laboratories for over a century. In essence, electrochemical processes within the battery have unique electrical and spectral (i.e., frequency) signatures that can be sensed externally. There are other methods of measurements, for example, using acoustic waves to assess, or even image, the materials within the battery.
It is the role of battery intelligence paired with analytics to make measurements, convert them into meaningful model inputs and combine them with non-linear physical/electrochemical models. For this to be effective and efficient, specific criteria must be observed.
First, measured inputs must be sufficiently frequent to represent real-life usage. In a battery, this could mean making measurements approximately every second. Second, the measurement instrumentation must be practical and dependable. For example, attaching a bulky and expensive laboratory instrument to the battery is not practical. This is the primary example where science and engineering intersect.
Next, the computational footprint must also be practical and dependable. Attaching a supercomputer to a battery is – unlike weather forecasting – not practical. Naturally, in our modern age of internet connectivity, there is a trend to move computation into the cloud. Depending on the application itself, the cloud may create several difficulties: data measurements become less frequent and slow to collect; data connectivity becomes expensive risking practical limitations; and scalability to a large number of batteries becomes challenging.
This is particularly of importance in electric vehicles. Implementing battery intelligence and analytics in an EV requires efficient models that can run on limited computational hardware, and measurement instrumentation that resides on the vehicle with as little dependence as possible on internet connectivity.